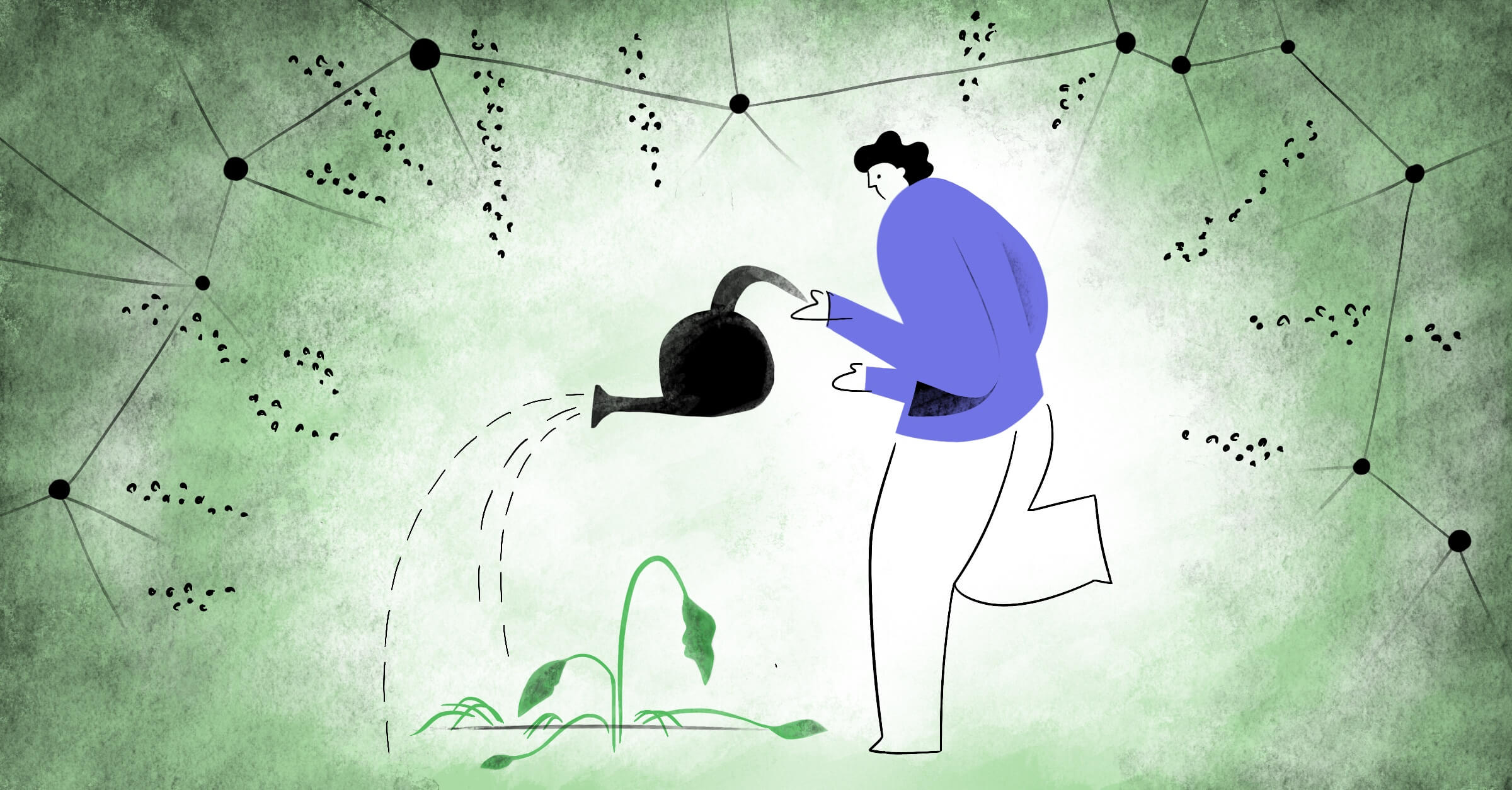
Data processing centres consume a vast amount of energy: about 1–1.5% of the world’s total consumption. Some companies have learned to sustain their operation through renewable and sustainable energy sources. However, the emergence of Large Language Models (LLMs) has complicated this task. This field requires about four times more energy than servers used for cloud applications.
The paradox is that while the new technology is intended to save labour and time resources, it is highly resource-intensive itself. The process of training and deploying an LLM model consumes a giant amount of energy and can cause serious harm to the environment.
The success of OpenAI’s ChatGPT large language model has sparked a race in the industry, during which tech giants have invested considerable sums in creating their own LLMs. AI’s carbon footprint is rapidly increasing due to its insatiable energy appetite and the carbon emissions associated with producing the equipment it uses.
A recent Massachusetts Institute of Technology study determined that training the most popular AI models has produced about 626,000 pounds of carbon dioxide. It’s quivalent to roughly 300 round-trip flights between New York and San Francisco.
And one data processing centre typically consumes energy equivalent to heating 50,000 homes per year.
According to researchers at OpenAI, since 2012, the computational power required to train the latest AI models has been doubling every 3.4 months.
Although the word “cloud” sounds intangible, it operates on quite tangible equipment: cables, fans, servers, routers, rare metals, and much more. Data processing centres used for cloud computing require significant amounts of energy for operation and cooling, leading to increased emissions of harmful substances.
It is worth noting that training an AI model is just the initial step, followed by its direct use, fine-tuning, or adaptation to other datasets. We mentioned above how much resources are needed to train one LLM.
Obviously, businesses primarily seek profit in savings. But sometimes, saving on development also means increasing eco-responsibility. Throughout 2023, we witnessed a race among tech giants, each introducing their own LLM models one after another. Behind the scenes of the loud announcements were huge expenses on computational power, which grew proportionally to the size of the model.
The AI wave swept over many other technology companies that were also considering creating their own LLM models. Fortunately, the widespread popularity of platforms such as Hugging Face has relieved them of the need to invest heavily in development. This platform enables the exchange and sharing of models and datasets among companies and research groups. In such cases, training proprietary models seems to be an inefficient use of resources.
And it’s not just about energy consumption for training LLMs from scratch. Open-source large language models developed by the community can be trained on a broader set of already filtered data. This data is cleaned of incorrect, outdated, or unwanted information and has better generalisation ability – making predictions on new, previously unused data.
When training your own LLM, there is a risk of overfitting the AI model to the primary data. This can lead to a deterioration in its generalisation ability and the quality of conclusions. It happens when the model memorises “noise” or unnecessary details in the training data too accurately, ultimately “losing sense” during reasoning.
With the active development of open-source LLMs, we observe an increase in small custom models created for specific industries and tasks.
This trend is becoming more noticeable. For example, the Harvey model specialises in creating LLMs for large legal firms. Character AI and Ava are designed to create digital companions.
Custom models can be optimised for specific tasks and datasets, allowing the use of fewer computational resources to achieve desired results.
Fortunately, many of the world’s major technology companies are making conscious efforts to reduce carbon dioxide emissions, use renewable energy sources, and minimise waste. For instance, Google invests in new technologies that, through machine learning, can cool its data centres using intelligent energy-saving thermostats.
Meta has committed to achieving zero emissions throughout its value chain by 2030. Microsoft recently hired a director to accelerate nuclear developments and implement a strategy using small modular reactors and micro-reactors to power Microsoft’s data centres.
The trend toward sustainable development in AI is evident, and companies involved in this area will find it increasingly challenging to ignore this aspect. After all, the main goal of AI is to improve our lives.
The human brain can do amazing things with low energy consumption. How do we create an artificial intelligence that functions in the same way? We still have to find the answer to this question for the harmonious and energy-efficient coexistence of machines and humans.
Have an AI implementation project in mind but don’t know where to start?