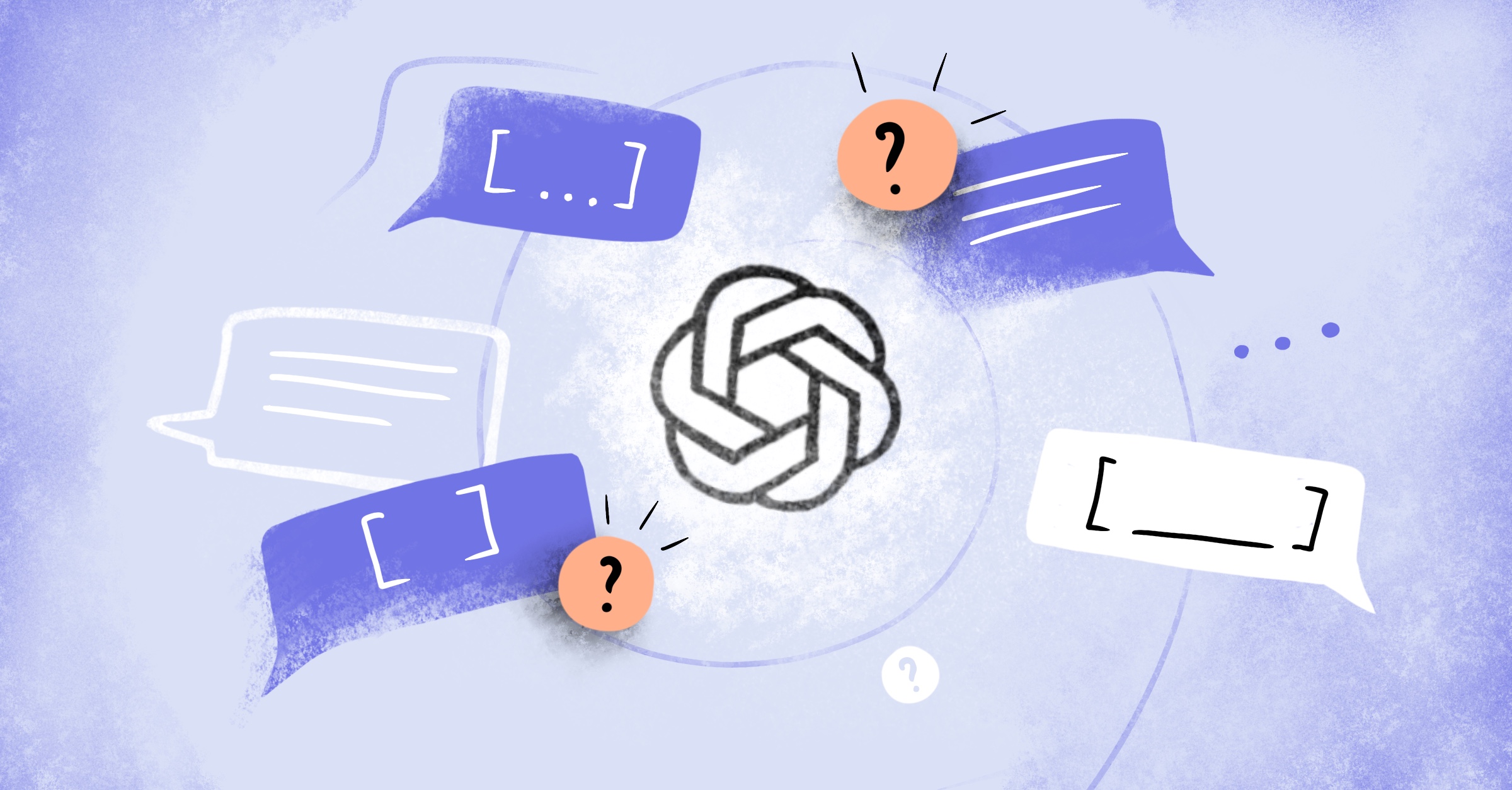
How to write proper prompts for ChatGPT to get the most accurate results when working with neural networks? This post will discuss methods for crafting the best prompts to obtain high-quality information from AI models and prevent hallucinations.
Recently, neural networks, especially ChatGPT, have become versatile AI assistants accessible to any user. However, to receive accurate answers, you must provide the neural network with as much context as possible, which means mastering a high-quality prompt.
The more detailed the prompts are, the better the neural network performs with specific information. Large Language Models (LLM) like GPT-3.5 consist of vast data. Without precise prompts, the neural network may struggle to grasp the nuances of the question and sometimes produce incorrect or irrelevant responses, leading to hallucinations.
First, let’s outline the limitations of ChatGPT:
1. It does not have knowledge of events or data that occurred after 2021 because it is trained on a vast database with updated information only up to that year. You can install the Bing AI extension based on ChatGPT in the Microsoft Edge browser to enable real-time internet data access.
2. ChatGPT has been trained on data from various languages, but most of its training material is in English. It is better to interact with it in English for more accurate responses and then translate the answer if needed.
3. ChatGPT has a token limit (words or characters) for each request or response. The limit varies depending on the version used: 4000 tokens for ChatGPT-3.5 and 8000 tokens for ChatGPT-4.
4. If you have exchanged much information during the conversation with the AI, we advise you to start a new chat to avoid potential issues with subsequent responses.
5. Due to high traffic, ChatGPT might be busy most of the time, and you may have to wait for a response. Consider subscribing to a premium plan if you require a faster response time.
Shot prompting techniques for AI vary in complexity: from simple phrases or questions to texts consisting of multiple paragraphs. The less effort you put into formulating a prompt for ChatGPT, the less effort the AI invests in it. “Zero-shot” prompt results are often unsatisfactory because the AI has to make too many decisions.
Zero-shot prompting is a mechanism AI uses for auto-completion, where the model gets complete freedom of action. In such cases, expecting a clear and structured answer is not recommended.
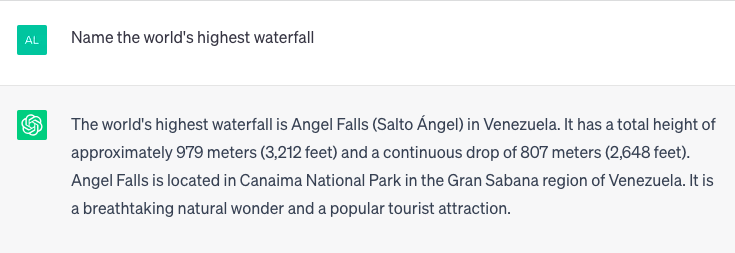
One-shot prompting means giving the AI an example of the desired outcome. A one-shot prompt is used to generate text in natural language with limited input data, such as a single example or template. This type of prompt is helpful when you need a specific format for the response.
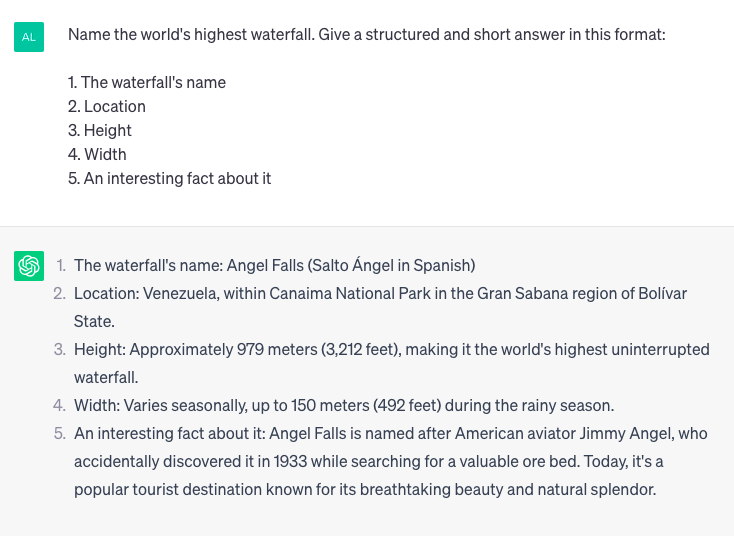
Few-shot learning is a prompt technique in which models are given a small number of examples, typically ranging from two to five, to quickly adapt to them.
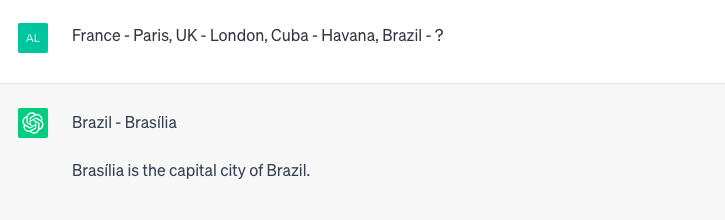
One of the most significant challenges with generative AI models is hallucinations. This term describes the phenomenon when a neural network produces results that do not correspond to reality, any given data, or any other identifiable pattern. AI tends to hallucinate more often when it lacks sufficient information to respond to your query.
What are some other reasons behind AI hallucinations?
Probabilistic nature. Generative models like GPT are based on probabilistic methods, which predict the next token, i.e., word or symbol in a sequence, considering the context. They assess the probability of each word and select the next one based on these probabilities.
This sampling process can sometimes lead to unpredictable and implausible outputs as the model might choose less likely words or phrases, resulting in hallucinations. ChatGPT is not trained to say “I don’t know” when it lacks information. Instead, it outputs the most probable response.
Lack of reliable information. Most language models cannot fact-check their output against a verified source in real time since they don’t have internet access. It makes it challenging for models to verify information for accuracy.
The complexity of the model. Modern generative models like GPT-3.5 have billions of parameters, which allow them to capture complex patterns in data. However, this complexity can also lead to overfitting and memorising irrelevant or false patterns, causing hallucinations in the generated responses.
AI can produce convincing and realistic hallucinations that can deceive people and lead to the spread of false information.
The main methods to counteract system hallucinations are related to engineering prompts—assigning AI a role, providing context and constraints, specifying the tone of voice, etc.
However, for solving complex tasks, these methods may not be sufficient. More sophisticated prompting structures like the Tree of Thoughts can be used in such cases.
The Tree of Thoughts is a powerful prompt writing method. It operates as follows: the original task is decomposed into components that the system unfolds and analyses independently. In other words, the model breaks down the problem-solving process into smaller steps or “thoughts,” making them more manageable.
Working on each component becomes an intermediate step towards solving the initial complex problem. This approach allows the neural network to consider several different lines of reasoning or approaches to solving the task.
An example could be a prompt in which three experts discuss a question, share their thoughts, and find the most optimal solution. The question is, “How to start an AI-based startup?”
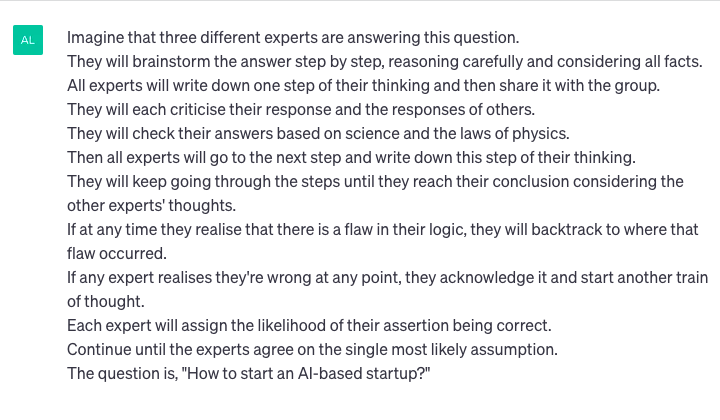
It seems that the model’s reasoning process starts as usual. Still, during its contemplation, the model weighs the pros and cons of each statement and provides additional information, building on its own insights.
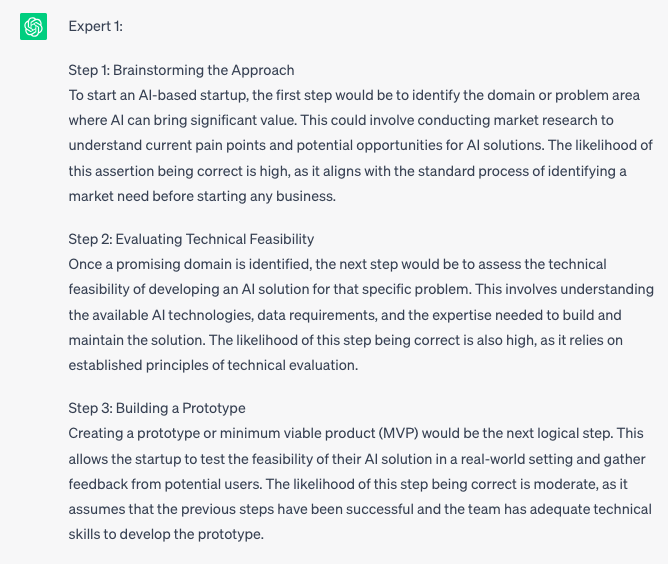
Next, the conversation involves the second expert, who, also building on the reasoning of the previous expert, continues to respond to the main question.
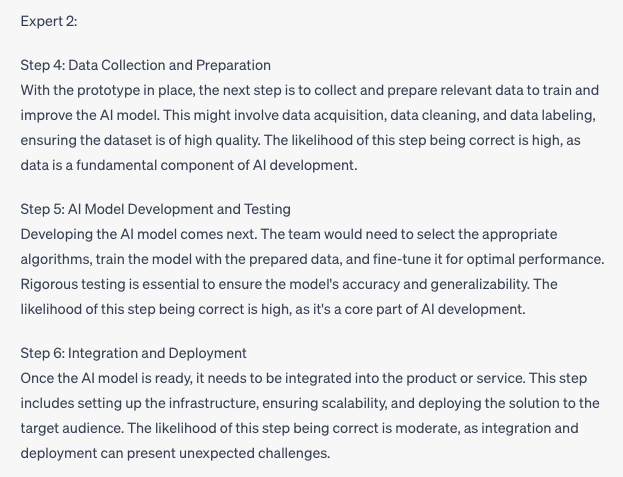
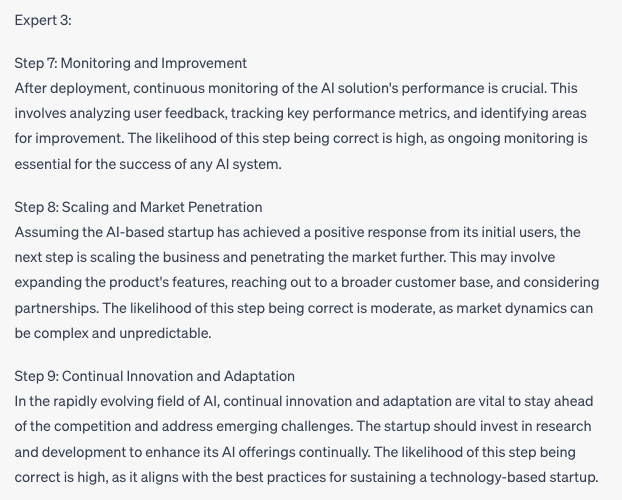
The reasoning will continue until the model finds the most optimal option for the final answer.
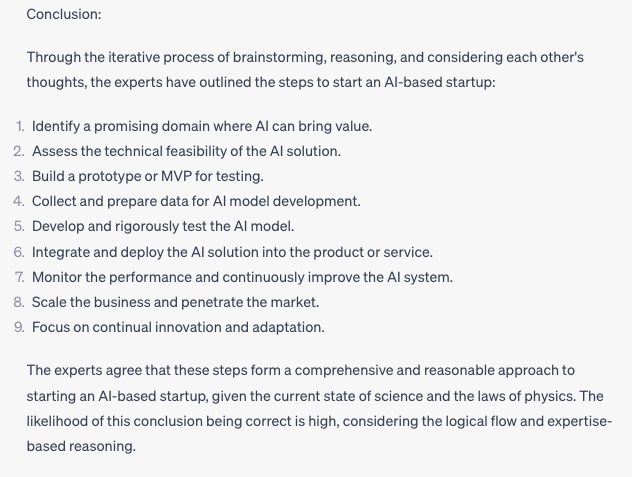
After considering the question from all angles and engaging in a detailed discussion of each step, the model arrives at a general conclusion that helps finalise the information obtained during the reasoning process.
The structure of the Three of Thoughts is designed to expand the capabilities and address issues of language models by providing a more flexible and strategic decision-making approach. The reasoning will continue until the model finds the most optimal option for the final answer.