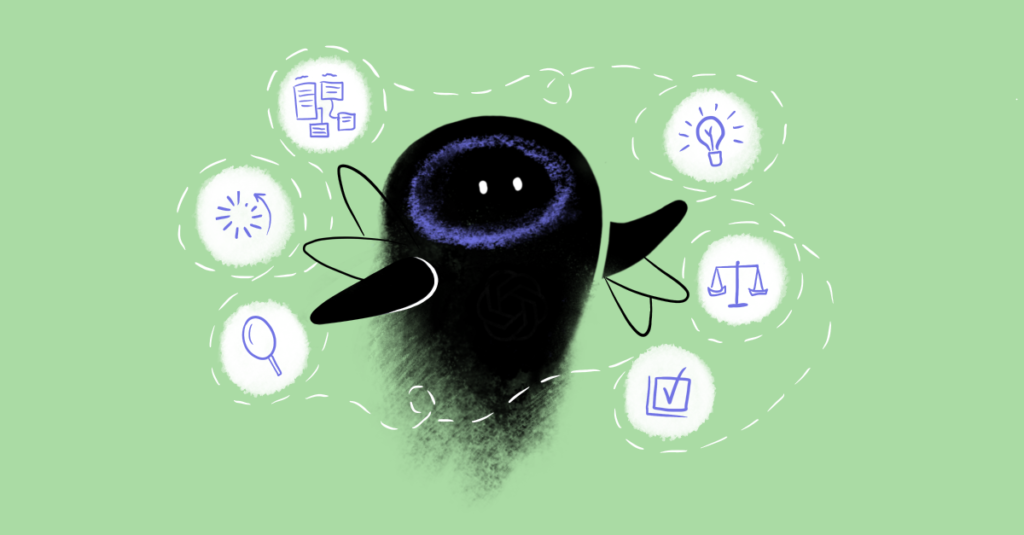
AI has made tremendous strides in recent years, and the next frontier, “Agentic AI”, promises even more exciting advancements. Unlike traditional systems that operate within predefined limits, Agentic AI envisions machines with a greater degree of autonomy. These systems could evolve to anticipate challenges, adapt to changing environments and find solutions in ways that go beyond simply following instructions. While we are still in the early stages, the potential for this shift is huge. Agentic AI has the power to transform how we approach problem-solving, driving innovation and efficiency in countless industries.
From optimising logistics under unpredictable conditions to personalising healthcare in real time, Agentic AI can handle complexity with finesse. But what exactly sets Agentic AI apart from its predecessors? How does it achieve this remarkable level of functionality? And most importantly, how can businesses and industries harness its potential to create smarter systems? This article explores the core principles of Agentic AI and its transformative impact across sectors. Let’s dive in and explore the AI of tomorrow today!
From self-driving cars to personalised healthcare assistants, the AI world has come a long way, but Agentic AI takes it a step further. This isn’t just about automation, it’s about creating AI that thinks, adapts and makes decisions in real time. Unlike standard AI which follows rigid rules or set scripts, Agentic AI operates with autonomy, learning from its environment and making independent choices while staying aligned with human-defined goals.
Therefore, one can say that the Agentic AI definition is a revolution within a revolution that is GenAI. As a rule, traditional systems heavily rely on human oversight: they execute preprogrammed tasks within a controlled framework. In contrast, Agentic AI balances out advanced cognitive capabilities with autonomy, which allows for quite intelligent responses even in novel and unpredictable situations. Advanced ML technologies like reinforcement learning and deep learning power this unprecedented adaptability. As a result, these systems can self-optimise and evolve over time.
While generative AI focuses on creating content whether text, images, or code based on learned patterns, Agentic AI goes a step further by taking autonomous actions to achieve specific goals. Unlike generative models that require human prompts, agentic AI LLMs can plan, make decisions and iterate based on feedback, adapting dynamically to complex environments. This shift from passive generation to proactive agency makes it a powerful tool for automation, problem-solving and decision-making across industries.
Quite predictably, in industries where adaptability is key, Agentic AI is already making waves. Take logistics, for instance. Standard AI might generate a delivery schedule, but Agentic AI takes it further. The technology anticipates roadblocks, recalculates routes on the fly and ensures packages arrive on time in any circumstances.
Similarly, in finance, Agentic AI algorithms monitor market trends in real time, dynamically adjusting investment strategies for maximum returns at minimal risks. In manufacturing, Agentic AI transforms production lines into smart systems that detect inefficiencies and predict equipment failures. This level of real-time adaptability is crucial in maintaining operational excellence.
Agentic AI in insurance streamlines claims processing by autonomously assessing documentation, detecting fraud and expediting approvals. Agentic AI automation enhances efficiency and ensures faster, more accurate policyholder support.
Dynamic problem-solving is yet another area where Agentic AI shines. In customer service, it handles routine queries, leaving human agents to focus on complex cases that require empathy and creativity. In healthcare, it’s not just crunching data it’s personalising treatment plans and adapting as new patient information becomes available. This kind of AI doesn’t just assist; it collaborates. For instance, Agentic AI can potentially monitor infection rates, recommend containment strategies and dynamically allocate resources like ventilators or hospital beds to areas with the greatest need.
Can it act without human intervention? Absolutely. Agentic AI is built to make decisions on its own, thanks to advanced learning models like reinforcement learning. These systems constantly refine their strategies, learning from every outcome to make better decisions next time. But don’t worry autonomy doesn’t mean a free-for-all. Safeguards ensure that its actions remain ethical and aligned with your goals.
One of the key features of Agentic AI is its ability to balance autonomy with control. Integrating ethical frameworks and human-in-the-loop systems ensures accountability and prevents unintended consequences. This makes it particularly well-suited for high-stakes environments like autonomous vehicles and critical infrastructure management.
While AI agents are software programs designed to perform specific tasks autonomously like chatbots or recommendation systems Agentic AI represents a more advanced concept. It not only executes tasks but also sets its own goals, adapts strategies in real time and operates with a higher degree of autonomy. Unlike traditional AI agents that follow predefined rules or workflows, Agentic AI can self-direct its actions, learn from experience and navigate complex, changing environments with minimal human intervention.
Traditional, rule-based AI systems stick to their scripts great for predictable scenarios but useless when things get messy. Agent-based systems like Agentic AI are different. They analyse, adapt and respond intelligently to the unexpected. Think of it like comparing a GPS that simply recalculates routes to one that predicts traffic jams and offers smarter alternatives before you even hit the road.
Agentic AI architecture mirrors natural decision-making processes. Each “agent” within the system operates semi-independently: it interacts with other agents and the environment to achieve complex objectives. This distributed intelligence approach enhances scalability and resilience, allowing the system to tackle multifaceted challenges with ease.
Functionality and design
However, the real breakthrough lies in Agentic AI’s ability to think and act independently while staying aligned with human goals. These systems don’t just execute tasks they interpret, adapt and respond to changing conditions in ways that traditional models can’t.
Goal setting and adaptability
How does Agentic AI set its goals? It’s not as simple as programming a checklist. Instead, these systems combine predefined objectives with real-time adaptability. For example, a logistics AI might start with a simple goal: deliver packages efficiently. But when faced with unexpected weather or traffic delays, it recalibrates, ensuring the task is completed with minimal disruption.
Agentic AI systems achieve this through multi-layered goal architectures. High-level objectives are broken down into sub-goals that are constantly reevaluated based on changing circumstances. This dynamic goal-setting mechanism ensures flexibility and precision, even in unpredictable environments.
Reinforcement learning is where the magic happens. By learning from experience, Agentic AI improves with every action it takes. Each success or failure feeds back into the system, refining its decision-making process. Think of it as a constant loop of trial, error and improvement whether it’s optimising energy use or guiding customer interactions.
What sets Agentic AI apart is its ability to generalise lessons across contexts. A reinforcement learning model trained on warehouse optimisation might apply similar strategies to streamline office workflows. This cross-domain adaptability amplifies its utility across industries.
Balancing autonomy and human values
Balancing autonomy with alignment to human values is no easy feat, but it’s the cornerstone of Agentic AI’s design. Ethical frameworks and clear boundaries ensure these systems stay focused on goals that matter like prioritising safety over efficiency in healthcare or fairness over speed in hiring decisions.
To achieve this, Agentic AI incorporates value-aligned algorithms that encode ethical principles into its decision-making processes. Techniques like inverse reinforcement learning and constraint satisfaction modelling help ensure that actions remain within acceptable ethical bounds, even as the system operates independently.
The key components of Agentic AI are what make this possible:
-
Sensory modules gather and process real-time data from the environment.
-
Decision-making engines analyse inputs and determine optimal courses of action.
-
Execution frameworks carry out decisions with precision and efficiency.
-
Learning modules continuously update knowledge and strategies to improve over time.
Handling uncertainty
And what happens when data is incomplete or ambiguous? Agentic AI doesn’t freeze up; it leans on probabilistic reasoning to fill in the gaps. For instance, a financial assistant might project market trends based on limited input, staying adaptable as more information comes in. Bayesian networks and other probabilistic models are often employed to manage uncertainty, enabling the system to make informed decisions even under constraints.
Risk and ethics
As promising as Agentic AI is, deploying systems with autonomy requires careful consideration of risks and ethical implications. These advanced systems are built to act independently, which raises questions about safety, accountability and alignment with human values.
Safety is a top priority in the design and deployment of Agentic AI. These systems rely on extensive testing, ethical frameworks and alignment protocols to minimise risks. However, their independence means they can face unexpected scenarios, making robust safeguards essential to prevent harmful outcomes. For instance, strict fail-safes and override mechanisms ensure human intervention is always an option when needed.
To enhance safety, developers use techniques such as adversarial testing and stress testing, where the system is exposed to extreme or edge-case scenarios to evaluate its responses. This rigorous approach uncovers vulnerabilities and ensures the AI performs reliably under diverse conditions. Additionally, multi-layered validation processes spanning from laboratory simulations to real-world trials help confirm the system’s readiness before deployment.
Agentic AI systems use reinforcement learning and advanced ethical programming to guide decision-making. This includes embedding moral and operational guidelines to prevent harmful actions. Regular audits, real-world simulations and transparent design practices help refine their responses, reducing the risk of unintended consequences.
A core strategy for preventing harmful decisions is the integration of “value alignment” algorithms. These algorithms ensure that the AI’s objectives are consistent with human ethical principles. For example, in healthcare, an Agentic AI assisting in diagnostics must balance speed with accuracy, prioritising patient safety above all else. Such priorities are encoded into the system through multi-objective optimisation, ensuring that ethical considerations are not sacrificed for efficiency.
One of the most pressing ethical concerns is bias. Like any AI, Agentic AI learns from data, which can inadvertently reinforce societal biases. Ensuring fairness requires diverse datasets, ongoing monitoring and collaboration with ethicists and sociologists to address these issues proactively. For instance, in hiring applications, biased training data could lead to discriminatory decisions. To mitigate this, organisations must conduct bias audits and incorporate fairness metrics into their evaluation processes.
Privacy is another key concern, especially in applications that handle sensitive data. Organisations must implement strict policies to protect user information. Techniques like differential privacy and federated learning are increasingly employed to minimise risks while preserving data utility. These approaches ensure that individual data points remain confidential, even as the AI learns from aggregated datasets.
The use of Agentic AI in surveillance also raises ethical red flags. Autonomous monitoring systems, if unchecked, can erode privacy and civil liberties. So, regulatory frameworks must enforce transparency and limit the scope of such systems to ensure they are used responsibly and proportionately.
When an AI agent acts autonomously, determining accountability can become complex. This is why it’s crucial to establish clear guidelines about responsibility before deployment. Developers, operators and organisations must share accountability through transparent documentation and predefined roles. Legal frameworks, like AI governance policies, are evolving to address this challenge.
One approach to ensuring accountability is the implementation of explainable AI (XAI). Explainability tools allow stakeholders to understand the rationale behind an AI’s decisions, making it easier to identify responsibility when things go wrong. For example, if an autonomous financial advisor recommends a poor investment, explainability tools can trace the decision back to specific data inputs or algorithms, facilitating accountability.
In industries with high stakes, such as aviation or healthcare, accountability measures are especially critical. Organisations must maintain detailed logs of the AI’s decision-making processes, enabling post-event analysis. Additionally, robust compliance mechanisms aligned with regulatory standards like GDPR or ISO help ensure accountability throughout the AI’s lifecycle.
Could Agentic AI become uncontrollable? While this concern may evoke dystopian scenarios, modern AI design prioritises alignment with human values. Through rigorous training and safety mechanisms, the likelihood of significant misalignment is minimised. However, continuous monitoring and adaptive learning updates are vital to ensure that the system’s goals remain aligned with the intended purpose over time.
One of the key strategies for maintaining control is the use of “safe exploration” techniques in reinforcement learning. These techniques limit the system’s actions to predefined safe boundaries, reducing the risk of harmful experimentation. Additionally, AI developers employ “impact regularisation,” which discourages the system from taking actions that could lead to significant unintended consequences.
Nevertheless, misalignment risks remain a topic of active research. Concepts like “AI corrigibility” are being explored to ensure systems remain receptive to human interventions, even after deployment. Corrigibility mechanisms allow humans to override or redirect the AI’s actions without resistance, safeguarding against potential runaway behaviour.
Beyond technical risks, Agentic AI poses broader societal challenges. Automation driven by autonomous systems can disrupt labour markets, leading to job displacement in certain sectors. While these technologies create new opportunities, it’s crucial to address the transition by investing in workforce reskilling and education programs.
There is also the risk of unequal access to Agentic AI, which could widen the digital divide between wealthy and underprivileged communities. Policymakers and organisations must prioritise equitable deployment to ensure that anyone can share the benefits of AI. Initiatives like open-source AI platforms and public-private partnerships can play a role in democratising access.
Mitigating the risks of Agentic AI requires a collective effort. Governments, industries, academic institutions and civil society must work together to establish global standards for ethical AI development. Collaborative frameworks, such as the Partnership on AI or the IEEE Global Initiative on Ethics of Autonomous and Intelligent Systems, provide valuable guidance on best practices.
International cooperation is particularly important in addressing challenges like autonomous weaponry or cross-border data sharing. By fostering dialogue and aligning regulations, global stakeholders can create a unified approach to managing the risks associated with Agentic AI.
Agentic AI is rapidly transforming how businesses operate, introducing dynamic capabilities that automate tasks and enhance decision-making. Whether it’s streamlining workflows or enabling personalised customer experiences, its applications are as diverse as the industries it serves. However, this revolutionary technology comes with practical concerns that businesses need to address to fully realise its potential.
Take logistics, for example. Agentic AI systems can optimise supply chain operations, dynamically rerouting shipments to avoid delays or minimising fuel consumption. These AI-driven systems analyse vast amounts of data, such as weather forecasts, traffic patterns and real-time inventory levels, to make decisions that were once the purview of human experts. By enabling predictive capabilities, Agentic AI reduces costs and improves efficiency across the board.
Agentic AI in healthcare powers virtual assistants that triage patient concerns, schedule appointments and provide personalised wellness recommendations. Imagine a scenario where a patient describes symptoms through a chatbot and the AI not only identifies potential issues but also schedules a consultation with the appropriate specialist. Such systems not only reduce the burden on medical staff but also enhance patient outcomes by expediting care.
Finance isn’t left behind either. Agentic AI identifies fraudulent transactions in real-time, analyses market trends and crafts tailored investment portfolios for clients. The speed and accuracy of AI in detecting anomalies and predicting market behaviours give financial institutions a significant edge, improving security and client satisfaction simultaneously.
Industries reaping the most benefits are those requiring adaptability and decision-making at scale. Retail, manufacturing and customer service are particularly ripe for transformation. For instance, e-commerce platforms deploying Agentic AI can curate personalised shopping experiences, using customer data to recommend products based on browsing history, purchase behaviour and even external factors like seasonal trends. This not only boosts customer satisfaction but also drives sales and brand loyalty.
Behind the scenes, building and deploying Agentic AI requires a strong technical foundation. Developers need skills in programming languages like Python, expertise in machine learning frameworks such as TensorFlow or PyTorch and an understanding of reinforcement learning principles. These technologies enable AI agents to learn from their environments and make decisions that align with predefined goals.
For effective implementation, businesses must also ensure robust infrastructure. Training and running Agentic AI often demand significant computational power, from high-performance GPUs to scalable cloud environments. This hardware supports the intensive data processing and model training required for AI systems to function optimally. Additionally, cloud platforms such as AWS, Google Cloud, or Microsoft Azure provide scalable solutions that allow businesses to deploy AI capabilities without investing heavily in physical infrastructure.
Integration and alignment with business goals
But it’s not just about hardware and code. Successful Agentic AI deployment hinges on aligning technology with business goals. Companies must identify specific problems AI can solve and set clear objectives for its implementation. For example, a retail company might focus on reducing cart abandonment rates through personalised recommendations, while a logistics firm might aim to cut delivery times by 20%.
Cross-functional collaboration is crucial in this process. Stakeholders from IT, operations and business units must work together to ensure that Agentic AI solutions meet practical needs while being scalable and cost-effective. Without this alignment, businesses risk investing in AI systems that fail to deliver tangible results.
Practical concerns also extend to ethical considerations. Agentic AI operates autonomously, making decisions that can have far-reaching consequences. For instance, an AI system in recruitment might inadvertently perpetuate biases present in training data, leading to unfair hiring practices. Similarly, a customer service AI might escalate disputes inappropriately if not carefully designed and monitored.
To address these concerns, businesses must implement robust ethical frameworks. This includes ensuring transparency in decision-making processes, regular audits of AI systems for bias and fairness and clear accountability mechanisms. In regulated industries such as finance or healthcare, compliance with legal standards adds another layer of complexity.
Despite its potential, deploying Agentic AI is not without challenges. High upfront costs can deter smaller businesses, especially those lacking technical expertise. Even for larger organisations, integrating AI into existing workflows can be a complex and resource-intensive process.
Data quality and availability pose additional hurdles. AI systems thrive on large, high-quality datasets. Incomplete, outdated, or biased data can compromise the performance of AI agents, leading to suboptimal or even harmful outcomes. Organisations must invest in robust data management practices, including regular updates, thorough cleaning and secure storage.
Another challenge is user adoption. Employees may resist AI adoption due to fears of job displacement or skepticism about its reliability. Effective change management strategies, including employee training and clear communication about the benefits of AI, are essential to overcoming these barriers.
Despite these challenges, the long-term benefits of Agentic AI far outweigh the initial investment. Businesses that successfully deploy AI systems report improved efficiency, enhanced customer experiences and increased profitability. For example, manufacturers using AI-powered predictive maintenance can reduce downtime and extend the lifespan of expensive equipment. Retailers leveraging AI for inventory management can minimise stockouts and overstocking, resulting in significant cost savings.
Moreover, Agentic AI fosters innovation by freeing human workers from repetitive tasks. This lets employees focus on creative problem-solving and strategic planning, driving business growth. Over time, the insights generated by AI systems can inform better decision-making at all organisational levels, creating a competitive advantage that is difficult to replicate.
From our experience in the evolving AI landscape, the future of Agentic AI lies in empowering human decision-makers rather than replacing them leveraging the strengths of both human intuition and machine intelligence. While automation has sparked fears about job displacement, the truth is far more nuanced. Most organisations recognise the value of human oversight in critical decision-making processes. Instead of replacing humans, Agentic AI is expected to complement their capabilities, driving more informed and effective decisions.
The idea that Agentic AI will replace human decision-makers outright is often overstated. Yes, Agentic AI excels at handling data-heavy tasks, analysing patterns and generating recommendations faster than any human could. However, there are nuances to decision-making ethical considerations, empathy and contextual understanding that AI cannot yet replicate.
Consider industries like healthcare or law, where decisions carry significant moral and societal implications. Here, AI can act as a powerful tool to augment human judgment. For example, a doctor might use AI to identify anomalies in diagnostic scans but would still rely on their expertise and patient knowledge to recommend treatments. Similarly, legal professionals may use AI for document analysis and research but retain control over case strategy and client representation.
The trend is clear: Agentic AI is poised to take on repetitive and data-intensive tasks, allowing humans to focus on areas where their creativity, emotional intelligence and ethical reasoning add the most value.
The next decade promises significant advancements in Agentic AI. One key area of growth is its ability to operate autonomously while remaining aligned with human goals. Advances in natural language processing (NLP) will enable more intuitive human-AI interactions, making these systems accessible to a wider range of users.
Improved reinforcement learning techniques will allow Agentic AI systems to adapt in real-time, learning from both successes and failures without requiring extensive retraining. This will make them more resilient in dynamic environments such as disaster response or cybersecurity.
Integration with emerging technologies like edge computing and quantum computing will further enhance Agentic AI’s capabilities. Edge computing will allow AI systems to process data locally, reducing latency and improving performance in applications like autonomous vehicles. Quantum computing, on the other hand, has the potential to revolutionise AI’s problem-solving abilities, enabling breakthroughs in fields such as drug discovery and supply chain optimisation.
Agentic AI and Artificial General Intelligence
Agentic AI could serve as a stepping stone toward Artificial General Intelligence (AGI). While current AI systems are designed to excel in specific tasks, AGI aims to replicate the versatility of human intelligence, enabling machines to perform any intellectual task that humans can do.
Agentic AI systems are already demonstrating traits that could inform AGI development, such as autonomous decision-making and contextual adaptability. For instance, multi-agent systems where several AI agents collaborate to achieve complex goals mirror some aspects of human teamwork and problem-solving. These advancements provide valuable insights into how AGI might be structured and trained.
However, AGI remains a long-term goal and the path toward it raises questions about safety, control and ethical considerations.
Regulations and Agentic AI
As Agentic AI becomes more integral to business and society, regulatory frameworks will play a critical role in shaping its development and deployment. Governments and industry bodies are already drafting guidelines to ensure AI systems are transparent, accountable and fair.
For example, the European Union’s AI Act seeks to categorise AI applications based on risk levels, imposing stricter requirements on high-risk systems like those used in healthcare and finance. Similar initiatives in the U.S. and Asia aim to balance innovation with safeguards against misuse.
Regulations are likely to focus on data privacy, algorithmic transparency and bias mitigation. Businesses adopting Agentic AI will need to prioritise compliance, conducting regular audits and implementing ethical design principles from the outset. Far from stifling innovation, these measures can build public trust and encourage broader adoption of AI technologies.
Collaboration between Agentic AI and humans
The true power of Agentic AI lies in its ability to collaborate with humans, creating synergies that neither could achieve alone. By automating routine tasks, AI frees up human workers to focus on creative and strategic initiatives. In customer service, for instance, AI can handle repetitive inquiries, allowing human agents to dedicate more time to complex issues that require empathy and critical thinking.
This collaboration is already transforming workplaces. Take project management platforms that integrate AI for task prioritisation and resource allocation. These tools not only improve efficiency but also provide teams with actionable insights, helping them make better decisions.
In the creative industries, AI is being used as a co-creator, generating ideas and prototypes that inspire human designers and artists. For example, AI can suggest architectural designs based on specific parameters, which architects can then refine and personalise.
In the future we can expect deeper collaborations between humans and AI. Hybrid teams, where human and AI agents work together seamlessly, could become the norm across industries. Training programs will evolve to include AI literacy, ensuring workers can effectively interact with and leverage AI tools.
The future of Agentic AI is not about domination but collaboration. By taking on tasks that are tedious or data-intensive, AI enables humans to focus on what they do best innovating, empathising and making complex decisions. Regulations and ethical frameworks will play a vital role in ensuring this collaboration is productive, equitable and beneficial for all stakeholders.
As we look to the next decade, the question is not whether Agentic AI will replace human decision-makers but how we can harness its potential to augment human capabilities. By addressing practical concerns and fostering collaboration, we can unlock a future where humans and AI thrive together.
Agentic AI stands at the forefront of technological innovation, poised to redefine how we work, solve problems and make decisions. Its potential lies not in replacing human intelligence but in amplifying it, creating opportunities for collaboration that were previously unimaginable. As this technology evolves, it will challenge us to rethink traditional workflows, redefine roles and embrace new ways of interacting with machines.
The road ahead is both exciting and complex. Regulations will ensure responsible development, ethical frameworks will guide its use and ongoing advancements will push the boundaries of what Agentic AI can achieve. The next decade will be defined by how effectively we integrate this technology into our lives and businesses not as a substitute for human ingenuity, but as a powerful partner.
By focusing on collaboration, transparency and adaptability, we can unlock the true potential of Agentic AI, ensuring it becomes a cornerstone of progress in a rapidly changing world. Together, humans and AI can pave the way for a future where innovation and empathy go hand in hand, transforming challenges into opportunities for growth and discovery.
Transform your business with Agentic AI!